4 out of 5 Americans are in favor of Remote Patient Monitoring!
Sounds exaggerated, doesn’t it? But that’s exactly what we are experiencing with the advancements in RPM technology.
In this blog, let’s witness the future with predictive analysis in remote patient monitoring and how it can enable us to witness the future!
How is Predictive Analytics Transforming Remote Patient Monitoring?
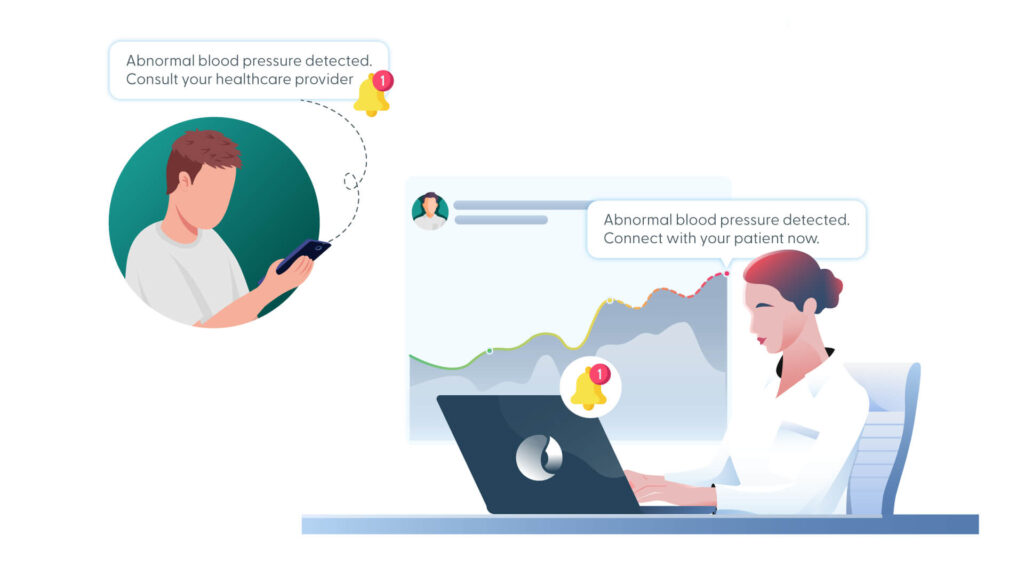
Riding on the waves of clinical patient data, the role of data in healthcare practices has improved diagnosis and treatment to a great extent. But what potential does predictive analytics have in transforming RPM?
Unveiling the Power of Predictive Analytics
The basis of the Remote Patient Monitoring program is data and it has played a very critical role in the advancements of the program and taking it to digital practice. Since a vast amount of data is being collected through these RPM programs, the application of predictive analytics can change the face of practice, turning it from reactive to proactive.
Download Whitepaper - The Power of Predictive Analytics in RPM Programs
Download nowKey Considerations for Implementing Predictive Analytics in RPM
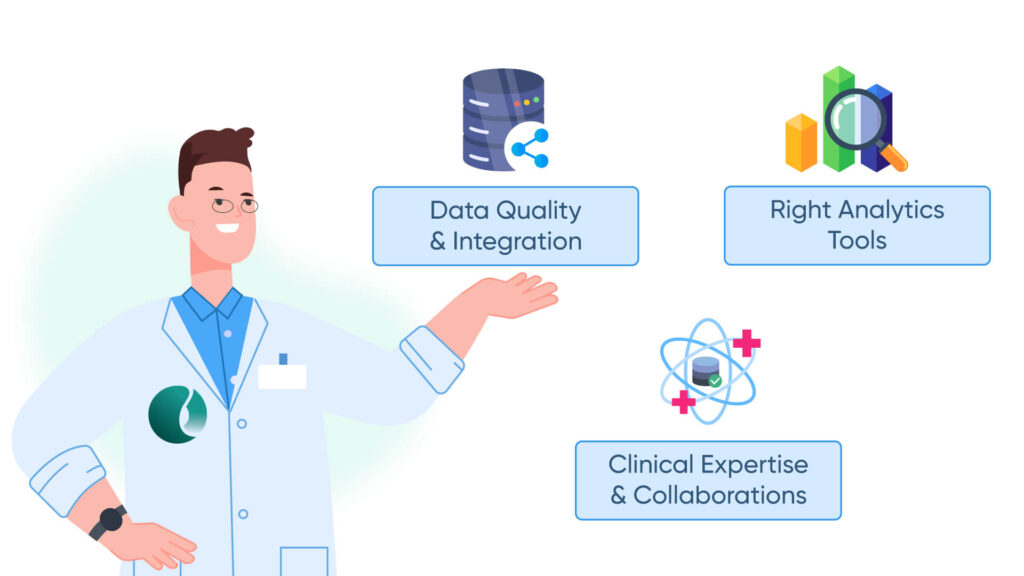
1. Data Quality & Integration
2. Choosing the Right Analytics Tools
3. Clinical Expertise & Collaborations
Optimizing RPM Programs with Predictive Analytics
Now that you’ve known what the requirements are for implementing in RPM programs, let’s see the remote patient monitoring benefits with predictive analytics.
1. Personalized Care Plans
2. Targeted Interventions
3. Improved Patient Engagement
Building a Future-proof RPM Program with Analytics
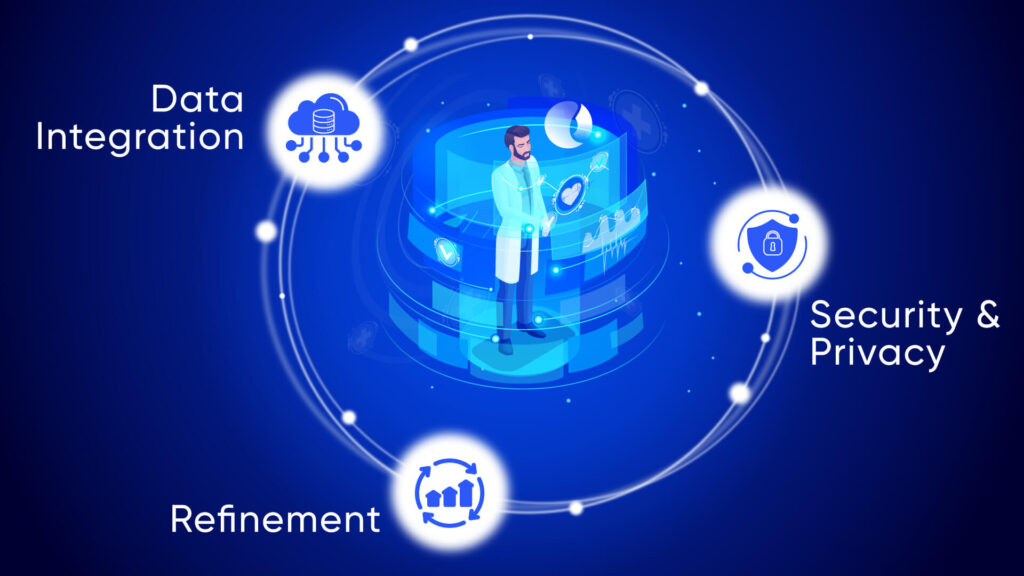
1. Data Integration & Infrastructure
Holistic care is one of the core aspects of remote patient monitoring programs. For this and instilling predictive analysis, data integrations are crucial to get a comprehensive and complete view of a patient’s healthcare journey. To enhance predictive analytics in the software, it is crucial to integrate RPM with other healthcare data sources, and for that, developing appropriate infrastructure cannot be ignored. Enabling healthcare interoperability with the right data integration pointers can enhance the overall journey and improve the accuracy of the predictive analytics model in RPM.
2. Security & Privacy Considerations
3. Continuous Improvement & Refinement
Remote patient monitoring with technology advancements is going to be more precise and contribute to enhancing patient engagement through predictive analytics. However, for a bright future of healthcare technology with predictive analytics, it is important to continuously improve the process. Since wider adoption will result in large amounts of data finding new patterns and trends in health, analyzing those with constant improvement in the models will improve the accuracy of suggestions, leading to improved patient outcomes.
Building a Successful Predictive Analytics RPM Program
1. Defining Program Goals & KPIs
While implementing predictive analytics in RPM programs, you should ask, ‘What is the purpose of this?’ This will help you define the program goals and develop important key performance indicators for measuring the success of the initiative.
2. Patient Engagement & Education
3. Ensuring Data Security & Privacy
Since we live in a world driven by information, data, and technology, especially in a remote patient monitoring program, which deals with a patient’s crucial health information, it is important to ensure data privacy and security.
Conclusion
As technology advances and given the evolution process of healthcare practices, predictive analytics in remote patient monitoring will become more relevant. Moving away from reactive healthcare to proactive healthcare services, predictive analytics will define the future of healthcare practices.
Furthermore, the potential of predictive analytics in improving patient outcomes and healthcare efficiency can literally change the way we see and approach care. However, healthcare providers must explore more options in predictive analytics and adopt the ones that ensure smooth healthcare delivery and efficient care practices.